Nonlinear Homogenization of Metamaterials for Neural Network-Based Material Modeling
In the realm of functional material design, achieving desired macroscopic properties through precise microstructural configurations remains a significant challenge. Numerical homogenization is a powerful computational approach used to derive effective macroscopic properties of materials with complex microstructures. By resolving the detailed microstructural features within a Representative Volume Element, this method enables the accurate prediction of material behavior under various loading conditions, bridging the gap between microscopic heterogeneities and macroscopic responses.
To avoid computationally expensive methods such as FE^2 which require treatment of boundary value problems across scales, sequential multiscale simulations can be applied. Thereby, a constitutive model is calibrated to homogenization data, which then acts as a surrogate model for the mechanical behavior of the microstructure. The homogenized behavior of microstructures is often highly nonlinear. Thus, we envision the use of physics-augmented neural network constitutive models [1,2]. These models combine the extraordinary flexibility of neural networks with a sound mechanical basis. By leveraging the detailed, high-fidelity data generated from homogenization simulations, we aim to develop robust and efficient predictive models that can accurately capture the complex behavior of heterogeneous materials within potential digital twin applications [3,4].
A Neo-Hookean-type material model with a Flory split is utilized to represent isochoric hyperelasticity in the COMSOL Multiphysics® Nonlinear Structural Materials Module. The RVEs consist of spherical and fiber inclusions with stiffness contrasts ranging from 10 to 1000, implemented through a user-defined, nearly incompressible material model. Efficient data sampling is achieved using strategically designed load paths to explore the material response comprehensively. This approach ensures that the neural network models are trained on a diverse set of stress-strain data, capturing the wide range of possible deformations and interactions within the microstructure.
Leveraging the capabilities of COMSOL Multiphysics®, our methodology encompasses the entire spectrum of homogenization, from linear to nonlinear regimes. Notably, our approach extends beyond traditional linear elasticity, incorporating nonlinear material effects of finite strain elasticity. This encompasses a modification of the Cell Periodicity Boundary condition. The homogenized first Piola—Kirchhoff stresses obtained from our simulations demonstrate high accuracy, validating the effectiveness of our approach. The results show that the nonlinear homogenization method can reliably predict the macroscopic stress response of heterogeneous materials under various loading conditions with low errors. The tangent stiffness is calculated using finite difference methods for each load step along the specified load paths. This step-by-step approach ensures a precise determination of the material's stiffness properties, which are crucial both for the homogenization process and for training advanced neural network-based models.
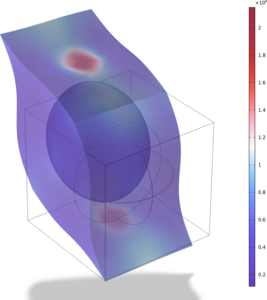
下载
- kannapinn_9131_poster.pdf - 1.79MB