Potential Fields Modeling to Support Machine Learning Applications in Maritime Environments
The integration of machine learning (ML) techniques into geophysical exploration and underwater mapping has emerged as a transformative approach for interpreting complex sensor data. This paper presents a comprehensive framework for using COMSOL Multiphysics to develop and validate potential fields models—specifically gravity and magnetics—in maritime environments. These models are designed to support machine learning applications, particularly for enhancing the predictive capabilities of uncrewed underwater vehicles (UUVs).
In the maritime context, accurate modeling of gravity and magnetic fields is crucial for detecting and characterizing underwater objects, ranging from low to high magnetic targets such as unexploded ordnance (UXO), shipwrecks, and geological features. We have developed a sandbox environment using COMSOL Multiphysics that allows for the precise creation and manipulation of complex geophysical sensing. This environment enables the detailed simulation of potential fields incorporating various target properties and environmental conditions, to generate synthetic datasets for ML training.
The modeling process begins with the definition of physical properties and the spatial configuration of the target objects. Low, medium, and high magnetic targets are systematically introduced into the simulation space, and their interactions with the surrounding medium calculated. The gravity and magnetic anomalies generated by these targets are then recorded from various UUV missions, providing a rich dataset that captures the nuances of potential field variations in different scenarios.
To validate our models, we employ a combination of theoretical predictions and empirical data. The theoretical framework leverages well-established geophysical principles, ensuring that the synthetic data aligns with expected physical behaviors. Empirical validation is conducted using field measurements from controlled maritime environments, where known targets are surveyed with high-precision gravity and magnetic sensors. The congruence between simulated and observed data confirms the accuracy and reliability of our models.
The validated models serve as the foundation for training advanced ML algorithms, with a particular focus on Long Short-Term Memory (LSTM) networks. LSTMs are well-suited for time-series analysis, making them ideal for interpreting the sequential data collected by UUV integrated sensors as they navigate underwater. The synthetic datasets generated from our COMSOL models provide diverse training examples, allowing LSTM networks to learn the complex temporal relationships and signature patterns associated with different target types.
In addition to LSTM, we explore other ML methodologies such as convolutional neural networks (CNNs) and ensemble learning techniques. Each method is assessed for its ability to accurately classify and predict sensor signatures under varying environmental conditions and target characteristics. By leveraging the robustness and versatility of COMSOL Multiphysics in combination with cutting-edge ML techniques, we aim to develop predictive models that significantly enhance the detection and characterization capabilities of UUVs.
Our approach demonstrates the critical role of validated potential fields modeling in supporting ML applications. The sandbox environment not only facilitates the generation of high-fidelity training data but also provides a controlled setting for testing and refining ML algorithms before deployment in real-world scenarios. The implications of this work extend beyond underwater exploration, offering a blueprint for integrating simulation-driven ML in various geophysical and engineering domains.
In conclusion, the fusion of COMSOL Multiphysics modeling with machine learning represents a powerful synergy, enabling the development of intelligent UUV systems capable of autonomously interpreting complex geophysical data. This research underscores the potential of advanced modeling and ML to drive innovation in maritime surveillance and beyond
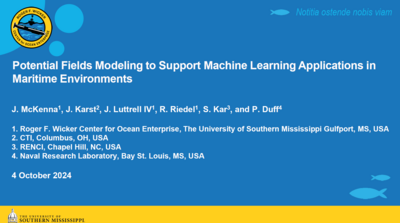